SAFETY DATASET FOR TRAINING AND VALIDATION OF AI PERCEPTION FUNCTIONS IN AD AND ADAS
Project B-0012 within SAFER's Open Research at AstaZero Program.
Goals and Purposes
The goal of this project is to evaluate crucial experimental properties and data collection configurations for establishing a safety dataset that can be used for AI training and validation of AD and ADAS.
AI, in particular, deep learning algorithms, are becoming the state-of-the-art technology used in AD and ADAS. However, the interpretability and reliability of these algorithms are known to be worrisome due to their data-driven and hence black box nature. This is not acceptable for automated systems deployed on the road, where guarantees on safety are required. Since these data-driven AI algorithms are to a large extent determined by the training dataset, it is very important to create a dataset such that it is fully aware of the safety requirements. Currently, available public data is limited to normal driving situations and data that covers edge cases like safety critical scenarios to test these systems is still lacking.
Results and effects
The outcome will be an initial version of a public dataset of driving scenarios relevant for safety studies, which contains ground truth labels on all sensor data that is ready-to-use for state-of-the-art AI algorithm training and validation. A particular focus shall be given to vulnerable road users (VRUs) as these traffic participants need thorough attention in L4/L5 systems and hence, a systematic methodology to establish a public dataset with safety-focused labeling is not available as of today.
The dataset will support researchers to have easy access and utilization of data to trigger research and development on safety aspects and improve the robustness of AI perception systems used in AD/ADAS. It can help in removing the last barriers for a wider introduction of AD and thus improve overall safety and contribute to the roadmap towards Vision Zero.
Planned Test Design and Implementation
An initial catalogue of five test scenarios that are relevant for global real life safety and the automated perception of dynamic objects (e.g. cars, trucks, pedestrians, cyclists) are selected and specified based on accident data, taking already covered use cases of ADAS systems into account. The specification of each scenario describes the pre-crash phase of an accident.
Each of the scenarios is driven in slow motion on the test track up to a point in time which is very close to the actual collision of the ego vehicle and the colliding dynamic object. The dynamic objects used in tests are real objects (e.g. no dummy cars) in order to mimic realistic sensor data of perception systems in real world usage. Variations of each scenario with different parameters (e.g. speeds, angles) are conducted.
A post-processing step will add labels to the data for training and validation of AI algorithms and transform the sensor data to real time speed of the collision scenario.
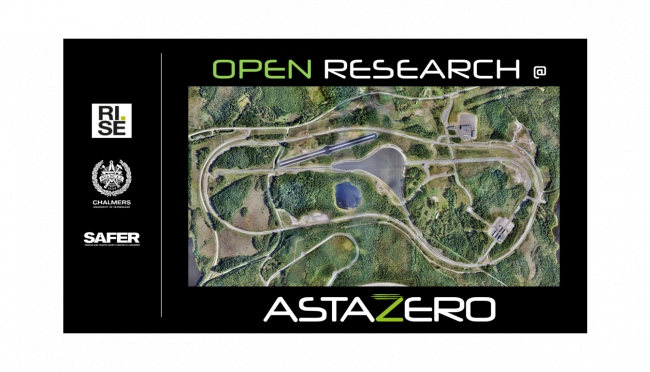