Jordanka Kovaceva's Doctoral thesis defence: Methods and models for safety benefit assessment of advance driver assistance systems in Car-to-cyclists conflicts
Welcome to Jordanka Kovaceva's Doctoral thesis defense: Methods and models for safety benefit assessment of advance driver assistance systems in Car-to-cyclists conflicts!
Jordanka Kovaceva is a researcher at Chalmers University, Division of Vehicle Safety since 2015. She has over 9 years’ experience working on naturalistic driving data using the largest naturalistic datasets in EU and US—euroFOT, UDrive and SHRP2. Her current interests include developing methods for analyses of traffic safety by using real world crash and naturalistic driving data.
- Opponent: Associate Professor Shan Bao, Department of Industrial and Manufacturing Systems Engineering, University of Michigan-Dearborn, Michigan
- Main supervisor: Jonas Bärgman (Associate Professor, Chalmers)
- Examiner: Marco Dozza (Professor, Chalmers)
Coffee and cake will be served after the defense. If you want to join this part, please let Sonja (sonja.laakso@chalmers.se) know.
Summary
To avoid traffic crashes, car manufacturers develop advanced driver assistance systems (ADAS) that can warn the driver or control the car. Since many cyclists die and get injured in road crashes every year, it is particularly important to develop and assess ADAS that help protect cyclists (one important example is car-to-cyclist situations). However, these systems are still far from perfect. The systems need to be properly assessed to understand their safety benefit and optimise their performance. The assessments are typically performed using virtual (computer) simulations and physical tests. This thesis looks at some ways to improve simulations: specifically, the three components of the virtual simulation of car-to-cyclist overtaking situations that were targeted in this research are driver models, vehicle models, and ADAS models.
The simulations and ADAS addressing car-to-cyclist overtaking situations require computational driver models to make important decisions—so this thesis developed them, by studying drivers’ everyday behaviour while they were overtaking cyclists. It also developed algorithms for model fitting (by making sure that the driver models give the same results as actual data collected from drivers) and for telling the ADAS when to intervene (so it is not so early it is annoying, and not so late that the crash happens anyway). Computing the ADAS intervention time accurately can be achieved by including detailed vehicle models. However, models with high level of complexity require high computation time, so we had to develop highly efficient algorithms for rapid safety benefit assessment. To improve ADAS models overall, a method was created so that data from both simulations and physical tests can be included in the safety assessment. (Different sources provide different information, so putting it all together is not straightforward.) Being able to use information from both sources means we can derive more accurate and robust conclusions regarding the safety benefit of ADAS.
The models and methods developed in this work can help developers get better ADAS onto the market; society will benefit from fewer crashes and cyclists’ injuries.
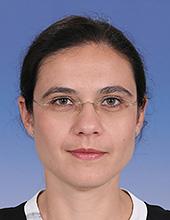