Crowdsourcing being tested as a tool for creating smart interfaces for self-driving vehicles
Can self-driving cars of the future be made better and safer with crowdsourcing? SAFER's researchers will now test this tool in their latest project ”Scale-up - Crowdsourcing for scaling up evaluation of external interfaces on automated vehicles”.
Imagine that you are a pedestrian and should walk across the street when a self-driving car approaches. In a normal traffic situation today, a quick eye contact with the driver would determine if you could safely cross the street. But what will happen in the future, when the car may be self-driving, without a human driver, or with a person behind the steering wheel that is not responsible for driving? How should you know that the car has seen you and will stop while you cross? The self-driving cars of the future will need a way to communicate their intentions with their surroundings. How this should be done in is now a focused task for SAFER's researchers.
“One of the major challenges we have is to verify that we find good solutions, that people really understand the vehicle. Conducting experiments on a test track or in traffic with people to gain knowledge how they perceive the car's signals takes a very long time and requires resources. Consequently, experiments are conducted on a small scale, which makes it difficult to generalize results. Finding more efficient ways to do evaluations of different interfaces would help us researchers a lot”, says Azra Habibovic, Research area director for Road user behavior at SAFER.
One way that SAFER's researchers are now investigating is whether it is possible to use online crowdsourcing to conduct experiments and evaluations of various interfaces with people from various parts of the world. By allowing a large number of people to participate in experiments online with a certain financial compensation, you can get valuable input to the research. The tool that will be used in this project is called Amazon M-Turk.
Azra tells more:
"In the current situation, however, we do not know if the results we get through crowdsourcing correspond to the results we would get if we met the people in reality. Perhaps you perceive the car's intentions in a different way when participating through a crowdsourcing function. The aim of our project is to understand, and if so, how an experiment in reality differs from an experiment performed by crowdsourcing".
The project will be part of SAFER’s portfolio within Road user behavior. It will be coordinated by RISE Viktoria with Volvo Cars (VCC) as partner and will run from 2019-03-01 to 2020-02-28 with a total budget of 1.000.000 SEK (500.000 SEK from FFI).
Research questions
The overall research question is: To what extent is the online crowdsourcing platform MTurk suitable for evaluation of external interfaces of automated vehicles (AV) as compared to controlled studies?
The specific research questions include:
1. Which design elements of an external AV interface can be evaluated via MTurk?
2. How do we design a study that will work both in MTurk and in a controlled experiment?
3. Which are the best qualitative and quantitative metrics for the studies?
4. Which participant selection criteria is suitable?
5. How to filter out inadequate data generated via MTurk?
6. What are similarities and differences between MTurk results and a controlled experiment?
More information about crowdsourcing
Crowdsourcing marketplaces make it easier for individuals and businesses to outsource their processes and jobs to a distributed workforce who can perform these tasks virtually. This could include anything from conducting simple data validation and research to more subjective tasks like survey participation, content moderation, and more. These tools enable companies to harness the collective intelligence, skills, and insights from a global workforce to streamline business processes, augment data collection and analysis, and accelerate machine learning development.
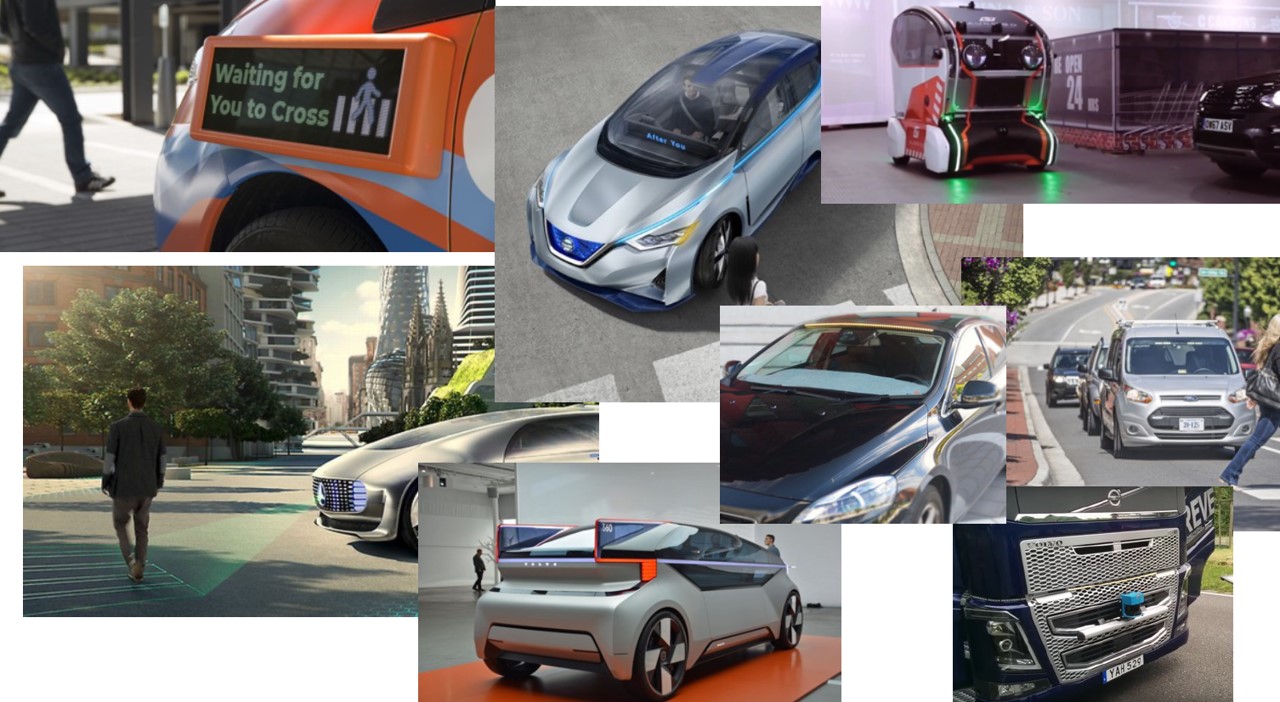