licentiate presentation of Rahul Pai: Is the risk worth the ride? Crash causation analyses of naturalistic e-scooter data
Welcome to the licentiate presentation of Rahul Pai: Is the risk worth the ride? Crash causation analyses of naturalistic e-scooter data!
Rahul Rajendra Pai is a PhD student in the Crash Analysis and Prevention Group, within the division of Vehicle Safety. His research focuses on the understanding of crash causation mechanisms and the infrastructural factors contributing to safety critical events on e-scooters. The research is mainly carried out using naturalistic data collected from shared e-scooters equipped with data loggers.
Rahul’s work was funded by Trafikverket within the SIMT project and supported by the data collection in e-SAFER.
- The discussion leader will be Annika Nilsson, Göteborg Stad
- Examiner: Jonas Bärgman, Chalmers
- Supervisors: Marco Dozza, Chalmers; Rikard Fredriksson, Trafikverket
More info about how to find the room: https://maps.chalmers.se/#10023cc2-2181-11e8-83e7-150e9dcc3277
If you want to join us on-line: https://chalmers.zoom.us/j/62228896792
Abstract
With the rapid increase in e-scooter popularity, there is growing concern about their safety with increased crashes, resulting in injuries and fatalities. The aim of this thesis is to investigate the key factors contributing to e-scooter crashes by analysing naturalistic riding data from instrumented rental e-scooters in an urban environment. Addressing the limitations of conventional crash databases, the two studies in this thesis used high frequency kinematic and video data to understand the behaviours and factors contributing to Safety Critical Events (SCEs; e.g., crashes and near-crashes). The studies focused on two topics: the identification of risk factors and the impact of methodological choices on risk assessment. This first-of-its-kind research used kinematic triggers to identify trips with at least one SCE, which were verified through video footages. The identified events were labelled to extract relevant variables related to the rider, infrastructure, environment, and trip characteristics.
The results from the studies highlight the critical need to adapt traditional definitions of crashes and near-crashes to reflect the unique characteristics of new forms of micromobility. The results also show the need to prioritize safety interventions based on both crash risk and crash prevalence. Specifically, factors such as riders using the e-scooter for leisure trips, the presence of intersections, trips taken on Fridays and Saturdays, pack riding, inexperienced riding were identified as significant contributors to increased risk in the decreasing order of prevalence. Therefore, countermeasures such as targeted safety campaigns, intersection with intelligent communication systems, hands-on training for new riders should be prioritized to have the largest impact on safety. The results also challenge certain assumptions derived from conventional crash databases, suggesting that factors like nighttime riding might not be as risky as previously believed, which raises questions about the necessity for nighttime bans. Methodologically, to identify risk factors from SCE, a reference for comparison is needed. This reference condition is often called baseline and captures typical riding scenarios where no SCE occur. In this thesis, two different approaches to baseline selection (random and matched) were used to explore the influence of baselines on crash risk and prevalence. The results indicate that both random and matched baselines are necessary to get the full picture of crash causation.
In conclusion, this thesis contributes to the field of micromobility safety by identifying the multitude of factors influencing e-scooter crashes and underlining the need for tailored definitions of SCEs. The insights form this thesis can guide the development of safety interventions, such as rider training programs, risky riding detection systems, and intelligent communication systems, to enhance e-scooter safety.
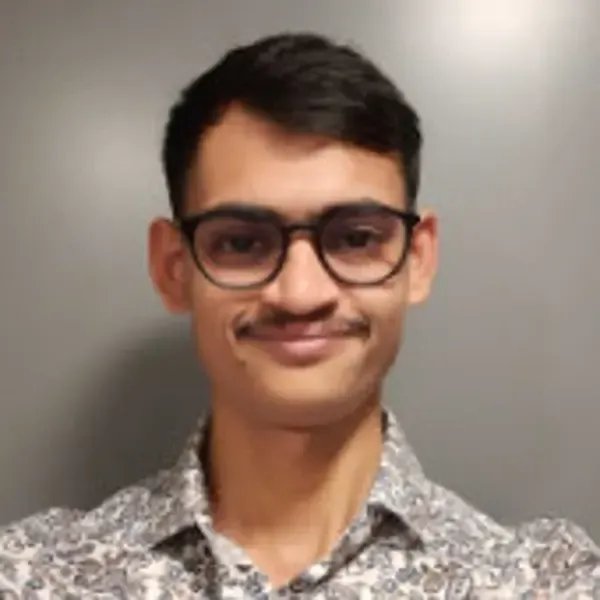