GUARD
GUarding Anonymization pRoceDures
The growing reliance on data-driven methodologies, particularly in machine learning, has significantly increased the importance of effective data collection practices. However, this process frequently involves the risk of capturing personal information, raising critical concerns regarding compliance with data protection regulations such as the General Data Protection Regulation (GDPR). Traditional anonymization techniques, such as image blurring, often compromise essential information—such as eye gaze, facial expressions, and the recognition of the presence of a face—thereby limiting the utility of the data for advancing data-driven innovation. In response to these challenges, the research community has increasingly explored generative AI techniques to develop attribute-preserving face de-identification methods. These approaches aim to balance privacy preservation with the retention of valuable data characteristics. Building on these advancements, the GUARD project investigates potential vulnerabilities in generative face de-identification processes that could lead to the leakage of true identities. Concurrently, the project seeks to mitigate these vulnerabilities by enhancing the robustness of de-identification models / algorithms and improving their capacity to detect and manage malicious inputs effectively.
Follow up project of MIDAS
Traffic safety benefit: Data is a very important asset for future mobility solutions and anonymization can provide new possibilities of collecting more data.
Key words: machine learning, XAI, anonymization, robustness, attack algorithms
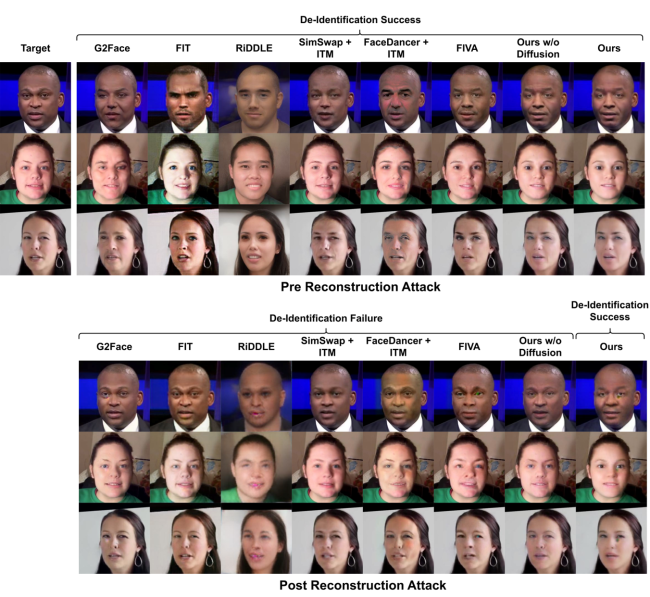