DEEP MULTIMODAL LEARNING FOR AUTOMOTIVE APPLICATIONS
This project aims to create multimodal sensor fusion methods for advanced and robust automotive perception systems. The project will focus on three key areas: (1) Develop multimodal fusion architectures and representations for both dynamic and static objects. (2) Investigate self-supervised learning techniques to the multi-domain data in an automotive setting. (3) Improve the perception system’s ability to robustly handle rare events, objects, and road users. The related applications are online mapping of the static environment, real-time object detection and tracking, and low-speed maneuvering.
The proposed solutions will be tested with real-life automotive data provided by the industrial partners, Volvo Cars and Zenseact. If the results are promising, they could potentially be incorporated into products, influencing the behavior of millions of vehicles.
The project promotes innovation and collaboration by bringing together prominent academic researchers from Chalmers University of Technology and leading industrial enterprises, Volvo Cars and Zenseact.
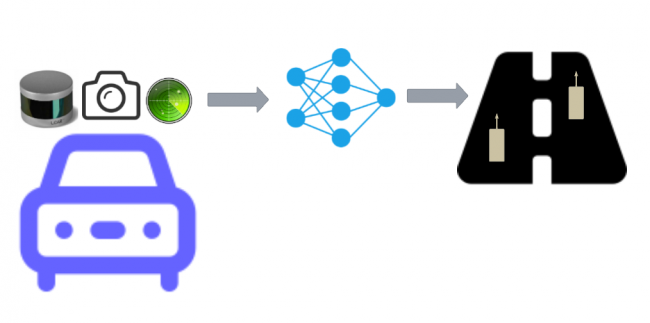