OpenBike-CD
Open data for Bicycle Classification for better planning and simulation, and for better Detection from motorized vehicles (OpenBike-CD)
Bicycling is an active mode of transport with positive effects on, among other things, health, physical conditions, less congestion, and less emissions. Attracting more users to bicycling is thus an important goal at the national and local government levels to achieve the Agenda 2030. Actions such as planning for bicycling-friendly cities, new bicycling infrastructure, improved maintenance are key to attract more users to bicycling. To be able to measure the impact of such interventions and adapt/adjust planning processes, it is important to follow-up the development over time of the bicycling flow/exposure over the entire bicycling road network, which must be done with a common and standardized method (Niska et al., 2010; Niska et al., 2012; Eriksson et al., 2019). Nowadays, it is even more crucial to follow-up the bicycling flow to adapt/adjust planning/maintenance processes, as more and more different types of bicycles of different characters and functionalities are sold and driven on the bicycle road network, e.g., standard bicycle, electric bicycle, box bicycle, tricycle, horizontal bicycle, and mopeds class 2. They are used by different types of cyclists (commuters, training bicyclist, school children) and passengers (toddlers) with different goals and needs. In addition, today's micro-mobility has changed the transport landscape with new type of vehicles such as electric scooters (shared e-scooters), electric standing boards (e-skateboards), inline skates, electric unicycles, segways and electric wheelchairs, which means that traditional bicycle flow measurements cannot interpret bicycle flow correctly.
The study aims to increase knowledge about bicycle flow by, together with industry, creating appropriate data for training algorithms (AI / ML) to distinguish (i) who is bicycling (gender, age), (ii) different type of bicycles (standard, electric, e-scooters, tricycles), (iii) route choice, and (iv) cyclists detection by motorized vehicles and to ensure bicycle flow data is quality assured and open to drive development at a faster pace and in the right direction according to Agenda 2030.
Purpose:
Create a database for training machine learning algorithms to:
1) Better understand bicycle flow by:
a) Classifying bicycle types such as standard, electric bicycles, e-scooters (how rides the bicycle).
b) Classifying cyclist sociodemographic characteristics, e.g., gender, age group (who rides the bicycle)
c) Detecting cyclists accurately and timely by (autonomous) motorized vehicles for developing cyclist-specific behavioral models.
2) Method development to standardize annotated open data for bicycle type classification, bicycle planning and simulation.
3) Follow bicycle flows (route choice) at important intersections by stationary camera stations to identify needs in terms of service and infrastructure standards on travel route choices.
Expected results:
Prepare a full application, build a consortium, define a budget, and apply for external funding to, for example, FFI/Vinnova and/or The Swedish Energy Agency.
Specifically, the pre-study will result in:
1. Well-defined data needs of stakeholders which will be the basis for industrial development of more accurate algorithms and better measuring equipment.
2. Well-defined how ‘raw data’ should be for the industry (i.e., bicycle and car), to be able to train and test ML algorithms, prototype and develop their equipment to the required data needs, see point 1.
3. Describe how the training/test data will be collected on the test sites by organizing an event with volunteers.
4. Describe research opportunities based on the data that will be collected and other bicycling data, being stored in an open database/data stock exchange.
5. Describe how cooperation is possible, among Nordic countries (e.g., NorBIKE).
6. VTI report on state-of-the-art. See Nordin (2022).
The results of the points above will be reported in the Final Report.
Implementation:
To gather data needs as well as row data types and data structure, a series of interviews and email questions, are/will be organized with relevant stakeholders from government, academia, bicycle, and car industry. Simultaneously, a series of Teams (Digital) meetings are/will be planned with key actors from the industry to get a deeper understanding of their data needs and data requirements.
International cooperation meetings are/will be planned as well to explore possibilities to cooperate among Nordic countries to make bicycling data available and open.
Data collection field tests are expected to be held, where each participant will be equipped with a chip/tag, where his/her data will be saved (Facit), and consent will be signed in advanced to meet GDPR requirements. In a first step, 3D video data will be collected to train algorithms to differentiate between bicycles and e-scooters. The possible test sites are listed below.
1. Motala
2. Oslo
The field test pilots will lay the grounds for further tests and studies, which will be part of a full application. For example, other type of sensors (lidar, infrared), classify other type of users (electric, moped, pedestrians), develop a standardized method to annotate bicycle data, develop models to predict bicyclists’ behaviour interacting with autonomous vehicles.
References:
Niska, A., Nilsson, A., Wiklund, M., Ahlström, P., Björketun, U., Söderström, L., Robertson, K. (2010). Metoder för skattning av gång- och cykeltrafik. Kartläggning och kvalitetsbedömning. VTI rapport 686, Statens väg- och transportforskningsinstitut. Linköping.
Niska, A., Nilsson, A., Varedian, M., Eriksson, J. och Söderström, L. (2012). Uppföljning av gång- och cykeltrafik. Utveckling av en harmoniserad metod för kommunal uppföljning av gång- respektive cykeltrafik med hjälp av resvaneundersökningar och cykelflödesmätningar. VTI rapport 743, Statens väg- och transportforskningsinstitut. Linköping.
Eriksson, J., Forsman, Å., Niska, A. och Karlström, J. (2019). Enhetlig metod för cykelflödesmätningar – en förberedande studie. VTI notat 16-2019, Statens väg- och transportforskningsinstitut. Linköping.
Nordin, L. (2022). Cykeldata - En översikt av data tillgänglig för cykelforskning i Sverige. VTI rapport (Utkast), Statens väg- och transportforskningsinstitut. Linköping.
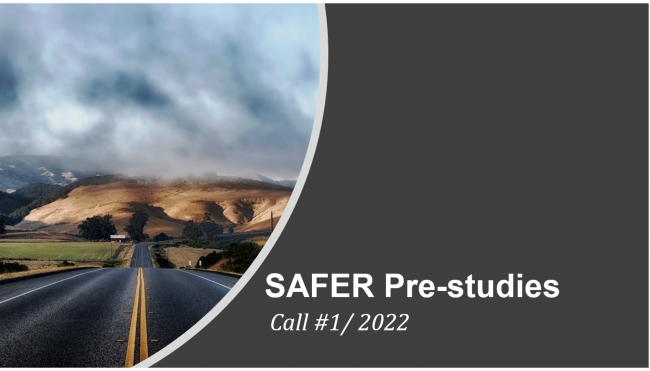